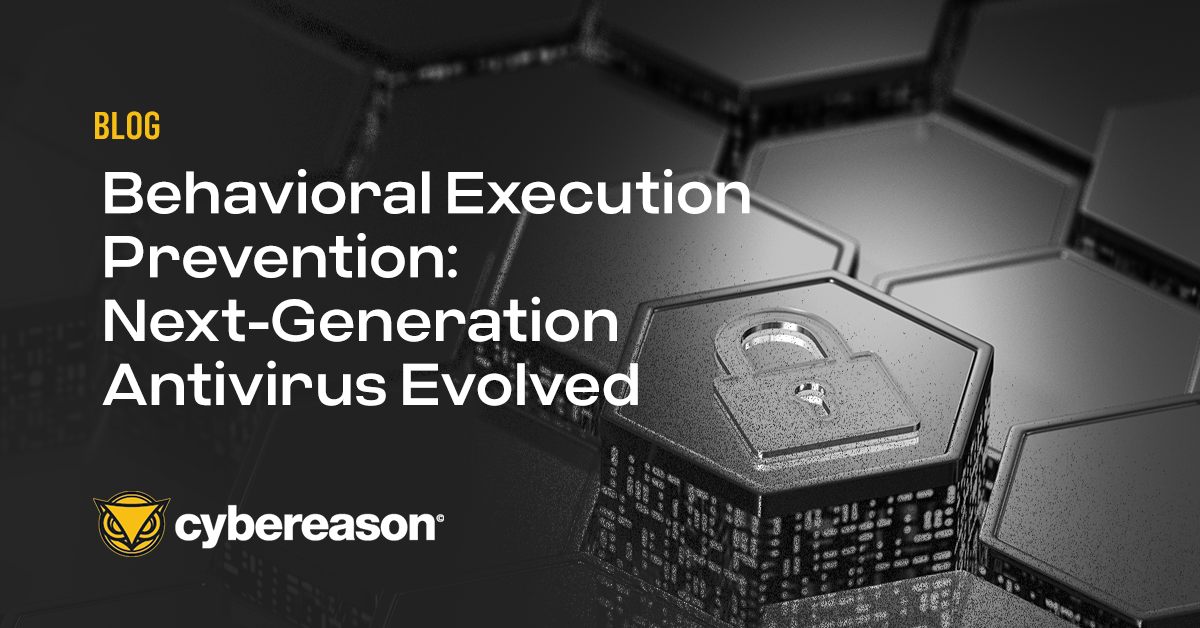
Behavioral Execution Prevention: Next-Generation Antivirus Evolved
Behavioral Execution Prevention stops threats posed by malicious actors who use trusted operating system software and native processes to conduct attacks...
Greg Day
Back in the 1990’s when I started at Dr. Solomons, my first introduction to threat intelligence was a one page table. It outlined all the common Boot Sector Viruses, the table mapping where the original boot or partition sector would be moved too, and which sectors the virus would write its code to. This was effectively our “encyclopedia” to help customers.
Typically they would call in after having run an antivirus on-demand scan that told them what virus was on their system. Our job would be to remotely verify that it was indeed that virus and then help the customer undo the damage by replacing the original clean sectors where they should be and removing any malware sectors. We also had a virus encyclopedia–both online and in hardback which showed at that stage the pace of new threats being developed.
If we could fix the problem, why did customers still need the encyclopedia? Even back in the day, many viruses had payloads that would trigger on specific dates or other programmable trigger points such as after a certain number of reboots.
Some payloads were obvious, overwriting the entire drive, others were far more subtle–for example, switching two random bytes of data periodically. The encyclopedia allowed compromised victims to verify if they needed to take additional steps.
Why look back in time? Things change. Through the late 1990’s, the volume of threats started to grow faster than researchers could manually analyze and document. To automate they used early sandboxing techniques, detonating suspected attacks on a mapped clean system where they could easily spot changes, allowing infection methods to be documented.
Basic attempts to find and trigger payloads were part of the process, but increasingly the description of payloads became more generic as there simply wasn’t enough time to complete full human analysis.
As volumes continued to explode, detection methods evolved to look for generic detection techniques, and I can’t tell you how many threats were covered using detection signatures that included Gen, or to use their full name “generic” detections:
These would be detections that could use a common method that was unique to a type of threat or a threat family–the value being you didn't need to have a unique detection for each threat which meant faster better coverage, but at the same time typically impact analysis would be skipped.
It took so much time and effort it started to just be generic auto-text descriptions, which was enough to understand how the threat worked, but all too often not what the actual payload was, and so other remediation steps were required.
Today security analysts leverage threat intel typically for two key purposes:
Not so long ago, many would pay to have access to good threat intel; however, in recent years, more and more has become freely available as the cybersecurity industry collaborates. The challenge is that they often don’t say the same thing about the same threat.
Take a simple example of VirusTotal: half of the detection engines tell you a threat has been found, the other half doesn’t. Which do you believe? Most likely, you believe that there is a threat–and that would be the cautious approach–yet, you would typically want to verify.
This would entail looking at the intelligence to find the attributes of the attack and confirming if they are on the systems you believe are compromised. If you find the common attributes, that's great; if not, typically an analyst doesn’t give up there.
They will keep looking to see if other vendors may have listed different attributes either for the same or other similar samples, and then they search again. It can be tough and time consuming to verify either a compromise or a false positive.
This has led to the introduction of Threat Intelligence Management (TIM) solutions that aim to do this enrichment on your behalf, reducing the time it takes an analyst to verify as it normalizes all the symptoms into one list. SOAR solutions can also achieve many of the same goals once you have written the lookup procedures against differing intelligence sources. It has the advantage of being able to also do some of the verification if you have programmed it to do so.
The challenge with threat hunting is the fidelity of the detection. How does a threat analyst have trust in the outcomes they get from the tools they use to find the problem–and of course, if they don’t trust it, which is too often the case–then how long does it take them to verify manually?
As a simple exercise, you can track the false positives of any cybersecurity tool you use. The detection levels of singular security capabilities can vary massively from low single digit percentages to very high detection efficacy. As such, knowing which of your tools and intelligence sources are behind them so you can trust the detections are high fidelity is key.
Some solution capabilities, including the Cybereason solution, now use machine learning Bayesian algorithms to continually monitor the efficacy of differing intelligence sources to weigh outcomes based on historical experience. Knowing how and why you trust an intelligence source can save a researcher literally hours a day.
All too often we over-pivot on finding the threat, and then rush to eradicate the threat itself. But in today's world where ransomware has gone through numerous evolutions–not simply just encrypting data, but analyzing it, downloading copies for resale or blackmail, stealing credentials to regain access the moment the actual threat is discovered and removed–it has become critical to truly understand everything each incident offers. Spending hours hunting for impact that never occurred can be as time consuming as finding the harm itself, the only blessing is the actual business impact is far less.
I’ve always liked the concept of CCTV for cyberattacks–the notion that we can use logs to play back the history of what happened. They, however, all too often fall foul of two issues: just like CCTV, they are triggered by “motion”--i.e. they only kick in when the threat has been detected, which in many instances isn’t actually when the threat first entered the premises and got a foothold.
Secondly, cameras usually only focus on one spot. Go to any bank and they will be awash with cameras in key areas as they want to track the adversary end-to-end. This all too often falls afoul of blind spots and takes “human” time and effort to jump between bits of footage from differing cameras.
The same principles apply in the cyber world, which is why so often it either takes tens if not hundreds of hours manually looking through logs to piece together the whole picture of what actually happened, and in so many instances you still end up with blind spots.
What's needed are dynamic cross-system (or as Cybereason calls it, cross-machine) correlations. This is at the heart of what we refer to as a MalOp (Malware Operation). It's the ability from any point in the process to track forwards and backwards across systems in your ecosystem to see what any threat actually has done.
It’s done through graphing all the relationships of events and interactions between systems (not just malware activities, all pre-filtered activities) in memory in the cloud to be able to build a real-time and look back perspective view.
Today, this insight comes from endpoint systems, but our journey to XDR in partnership with Google will enable a myriad of other security capabilities and vendor telemetry to be aggregated and correlated and then delivered. Not an easy task when–just like the old days of video–each uses its own structure and format, so must be first normalized before its data can be added into the digital picture.
Today, the biggest limiting factor in a Security Operations Center is its people. We have the ability already to produce way more security intelligence than can be processed. The challenge is knowing which data to trust, and then being able to knot it together through automation to both have confidence in the security alert being generated, but that really is only the starting point.
If you can’t easily understand what has actually happened, you can waste hours looking for nothing or take too long to react to something real whilst gathering evidence, thus allowing the scope of the impact to grow. As such, intelligence efficacy and the efficiency in which we can leverage it in a SOC must be at the heart of every cybersecurity strategy.
Cybereason is dedicated to teaming with defenders to end attacks on the endpoint, across the enterprise, to everywhere the battle is taking place. Learn more about Cybereason AI-driven XDR here or schedule a demo today to learn how your organization can benefit from an operation-centric approach to security for increased efficiency and efficacy.
Greg Day is a Vice President and Global Field CISO for Cybereason in EMEA. Prior to joining Cybereason, Greg held CSO and CTO positions with Palo Alto Networks, FireEye and Symantec. A respected thought leader and long-time advocate for stronger, more proactive cybersecurity, Greg has helped many law enforcement agencies improve detection of cybercriminal behavior. In addition, he previously taught malware forensics to agencies around the world and has worked in advisory capacities for the Council of Europe on cybercrime and the UK National Crime Agency. He currently serves on the Europol cyber security industry advisory board.
Behavioral Execution Prevention stops threats posed by malicious actors who use trusted operating system software and native processes to conduct attacks...
Advanced XDR doesn’t rely on a flood of non-contextual threat alerts from across disparate assets, but instead delivers deep context and correlations between assets to detect sooner and automates responses to mitigate faster...
Behavioral Execution Prevention stops threats posed by malicious actors who use trusted operating system software and native processes to conduct attacks...
Advanced XDR doesn’t rely on a flood of non-contextual threat alerts from across disparate assets, but instead delivers deep context and correlations between assets to detect sooner and automates responses to mitigate faster...
Get the latest research, expert insights, and security industry news.
Subscribe